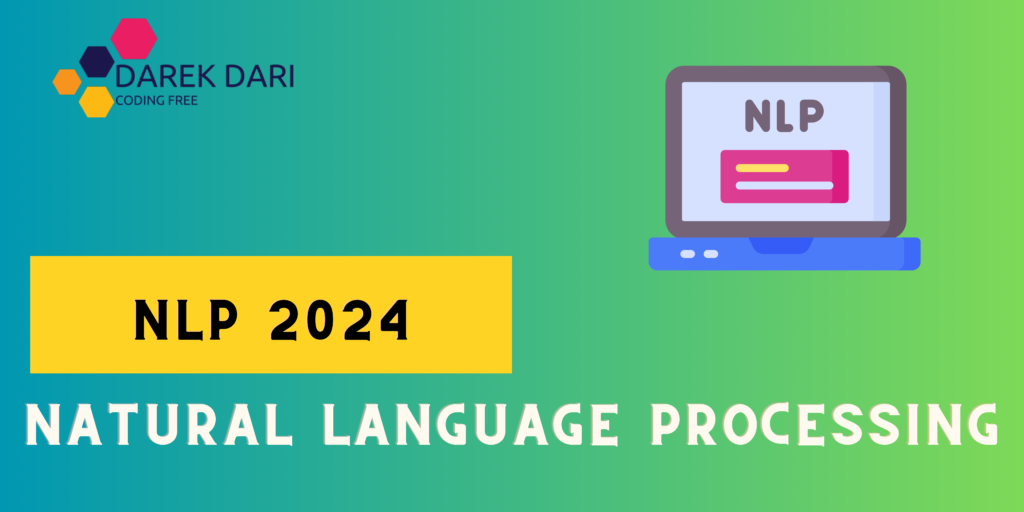
Table of Contents
Introduction to NLPππ€π
Hey there! Ever thought about how computers understand the crazy things we say? It’s not magic, it’s Natural Language Processing (NLP) – the coolest thing in the world of AI.
We’re talking about teaching machines to read, understand, and even generate text just like us! This isn’t your typical old textbook. We’ll explain NLP in a way that will make you go “wow, that’s awesome!”.
Get ready to learn about chatbots that can hold conversations, machines that can translate languages instantly, and algorithms that can write articles (maybe even this one!). If you’re interested in the future of AI and how it’s adapting to our language, then buckle up for an exciting journey!
We’ll dive into the basics of NLP, debunk some myths, and show you why this field is changing the way we interact with technology. Let’s get started!
π Who Is This Course For?
Are you a language enthusiast who has ever wondered about the intricacies of language comprehension by machines? This course is designed specifically for you, even if you have no prior coding experience.
Calling all tech enthusiasts! If you’re fascinated by the intersection of language and technology, we invite you to join us and discover how NLP is revolutionizing the future.
natural language processing ai
ai natural language processing
natural language processing in ai
what is an nlp coach
Attention students and professionals! Whether you’re a student looking to expand your knowledge or a professional considering a career change, this course provides a solid introduction to NLP.
π What Awaits You?
- Interactive Learning: Say goodbye to dull lectures! Engage in hands-on activities and exercises that breathe life into NLP concepts.
- Real-World Applications: Discover how NLP operates in everyday life, from virtual assistants to language translation.
- No Coding Fear: Don’t speak the language of code? No worries! We’ll gently introduce you to the basics, making NLP accessible to everyone.
what is llm in machine learning
neuro linguistic programming certification
neuro-linguistic programming certification
π Course Highlights:
- Text Adventures: Immerse yourself in the realm of tokens, corpora, and linguistic marvels through interactive text-based adventures.
- Code Playgrounds: Experiment with simple Python snippets to demystify the language of machines.
- Challenge Yourself: Confront real-world examples, solve problems, and unlock the potential of NLP in your own projects.
What is the natural language processing NLP?
Isn’t it amazing when your phone can quickly translate texts or a chatbot can answer your questions like a real friend? That’s the wonder of Natural Language Processing (NLP)!
Computers are great with numbers, but human language can be a confusing mess. NLP helps bridge that gap by teaching machines to understand the way we communicate. It involves reading, interpreting, and even generating text that sounds human-like!
NLP isn’t just something out of a sci-fi movie. It’s already changing the world around us. From chatbots handling customer service inquiries to social media platforms filtering out negativity, NLP is everywhere! Even the blog post you’re reading might be influenced by NLP algorithms (rest assured, a real human wrote itβ¦ for now).
llm ai
what is nlp
what is an nlp
nlp techniques
n l p techniques
If you’re curious about how computers are learning our language and why it’s so important, then stick around! We’ll be diving into the key concepts of NLP, debunking myths, and showing you why this field is the future of human-computer interaction. Let’s geek out!
What is natural language processing NLP solution?
We’ve been discussing NLP and how it enables computers to comprehend our complex human language. But what about NLP solutions? Get ready, because we’re about to break it down in a way that’s not filled with technical jargon.
nlp technique
neurolinguistic programming techniques
techniques of nlp
llm machine learning
nlp practitioner
neuro-linguistic
Think of NLP solutions as tools in a toolbox. Each tool addresses a specific issue related to how computers process language. Here’s the lowdown:
- Unlocking the Meaning: Some NLP solutions focus on sentiment analysis. Imagine a machine that reads social media posts and tells you whether people are feeling happy, angry, or somewhere in between. Pretty cool, huh?
- Translation Nation: NLP-powered translation tools can break down language barriers like never before. Think you can only speak English? NLP allows you to have conversations with people from all over the world.
- Text Summarization on Point: Ever find yourself overwhelmed by lengthy articles? NLP solutions can summarize the key points for you, saving you valuable time.
- Chatbots that Rock: Remember those clunky chatbots that couldn’t answer your questions? NLP is making them smarter, capable of holding conversations that feel natural (well, almost!).
neurolinguistics programming
nlp meaning
meaning of nlp
These are just a few examples, my friend. The possibilities with NLP solutions are limitless! They’re revolutionizing fields such as healthcare, finance, and even entertainment.
So, the next time you witness a machine understanding your language, remember that it’s not magic, but the power of NLP solutions at work! Stay tuned, because we’ll be diving deep into some of these solutions and how they’re changing the game!
What is NLP and its application?
Have you ever considered how your phone can instantly translate languages or how a chatbot can respond to your questions in a natural manner? That’s the magic of Natural Language Processing (NLP) at work!
NLP is like a language tutor for computers, helping them grasp the intricacies of human communication. It enables computers to understand, interpret, and even create text that mimics human speech.
NLP isn’t just a far-off dream; it’s already an integral part of our daily routines. From chatbots handling customer queries to social media platforms filtering out negativity, NLP is all around us!
nlp
natural language processing
large language models
neuro-linguistic programming
neuro linguistic programming
And the best part? NLP tools are like a treasure trove of technology that addresses various language-related challenges for computers. Here’s a sneak peek at what NLP can achieve:
- Sentiment Analysis: Picture a machine that can analyze online reviews and determine whether people love a product or are extremely upset. NLP uncovers the emotions hidden in words.
- Translation Excellence: NLP-powered translation tools are breaking down language barriers, allowing you to converse with people globally without needing a translation guide.
- Simplified Text Summarization: If you’re drowning in lengthy articles, NLP can condense them into concise summaries, saving you time.
- Chatbots with Character: Gone are the days of robotic chatbots; NLP is enhancing their conversational abilities, making them more human-like.
what is natural language processing
nlp models
nlp model
models in nlp
nlp training
training in nlp
nlp machine learning
This is just a glimpse of NLP’s capabilities. It’s transforming industries like healthcare, finance, and entertainment, opening up endless possibilities!
Is NLP an AI?
Hey there! We’ve given you the lowdown on NLP, explaining how it helps computers grasp our complex human language. But hold up, is NLP itself a form of AI? Well, it’s not a simple yes or no answer. Let’s dive into it.
Picture AI as the head honcho. It’s a vast field dedicated to developing intelligent machines that can imitate human abilities. On the flip side, NLP is a specific subset of AI. It zeroes in on a particular facet of intelligence: comprehending and processing human language.
nlp therapy
nlp in therapy
nlp therapist
nlp coach
nlp coaches
coach nlp
Here’s an analogy: Envision AI as a massive tech conglomerate with numerous departments. NLP would be one of those departments, specializing in communication and language technology. They collaborate to fulfill AI’s overarching objective of enhancing machine intelligence.
Here’s why NLP isn’t exactly AI:
- Focus: AI covers a wider range, including problem-solving, learning, and decision-making, not just language. NLP hones in on the nuances of human language.
- Techniques: AI employs various methods like machine learning and deep learning. NLP also uses these techniques but tailors them specifically for language-related tasks.
Therefore, NLP acts as a specialized instrument in the AI arsenal. It aids AI in reaching its objectives by enabling machines to comprehend and engage with us through language.
In essence: They’re partners in crime, not one and the same. AI is the big picture, and NLP is a vital tool that assists AI in grasping the world around it, particularly how we communicate.
nlp certification
certified nlp
nlp certifications
nlp certified
certification in nlp
nlp certificate
Is NLP AI or ML?
Alright, get ready! Let’s unravel the connection between NLP, AI, and Machine Learning (ML). It can be confusing, but we’ll explain it in a way that will make perfect sense!
Imagine a massive tech company:
- The Big Boss: Artificial Intelligence (AI) – This is the ultimate goal, creating intelligent machines that can imitate human abilities in various areas. Think problem-solving, learning, decision-making, and yes, understanding language.
- The Specialized Department: Natural Language Processing (NLP) – This is a specific branch within AI. It focuses on a crucial aspect – understanding and processing human language. NLP is like a team of engineers who design and develop the communication technology for the big AI company.
- The Toolbox: Machine Learning (ML) – This is a collection of tools and techniques used by many departments of the company, including NLP. ML allows machines to learn from data without explicit programming. It’s like equipping the engineers with a toolbox full of powerful learning algorithms.
Here’s the key:
- NLP is not exactly AI, but it plays a vital role in it. It helps AI achieve its goals by enabling machines to understand and interact with us through language.
- NLP heavily relies on ML. Those advanced ML algorithms in the toolbox are like the building blocks for NLP to function. NLP engineers use them to train machines to understand the intricacies of human language.
So, to answer your question:
- NLP is not the same as AI. It’s a subfield within AI with a specific focus.
- NLP utilizes Machine Learning techniques to accomplish its goals. It’s like using a specific set of tools from a larger toolbox.
Is ChatGPT NLP?
Yes, ChatGPT is undoubtedly an NLP (Natural Language Processing) system. It leverages large language models that undergo training on extensive volumes of text data.
This empowers ChatGPT to produce text of exceptional quality, facilitate language translation, compose diverse creative content, and provide informative responses to your inquiries. Essentially, it revolves around comprehending and interacting with human language.
How to use NLP?
NLP is not your typical social media app that you can use directly. It’s actually a fascinating field within computer science that encompasses a wide range of techniques and applications. However, if you’re interested in harnessing the power of NLP, there are several options available to you:
- Online NLP tools: There are websites and apps that provide NLP functionalities such as sentiment analysis, text summarization, and machine translation.
- Programming libraries: If you’re a programmer, you can utilize NLP libraries like spaCy or NLTK for Python to create your own NLP applications.
- Cloud-based NLP services: Additionally, there are cloud platforms like Google Cloud or Amazon Web Services that offer NLP services which can be seamlessly integrated into your own applications.
Why is NLP difficult?
Human language is a complex and messy form of communication. This is why NLP (Natural Language Processing) faces several challenges:
- Ambiguity: A single sentence can have multiple meanings depending on the context. NLP systems need to be able to understand and interpret these nuances accurately.
- Sarcasm and Humor: Computers struggle to grasp the subtle cues of sarcasm and humor in human language. NLP systems find it challenging to detect and understand these forms of expression.
- Constant Evolution: Language is constantly evolving with the introduction of new words, slang, and expressions. NLP systems need to adapt and keep up with these changes to accurately process and understand the language.
- Lack of Common Sense: Humans have a natural understanding of the world, which helps us interpret language based on common sense reasoning. NLP systems need to learn and incorporate this kind of common sense reasoning to improve their understanding of human language.
what are nlp techniques
what is nlp technique
what is nlp techniques
natural language processing models
Despite these challenges, NLP is a rapidly growing field with significant advancements. As technology progresses, NLP systems will continue to become even more sophisticated in their understanding and processing of human language.
How is NLP coded?
Here’s a basic code example of NLP using Python and the NLTK library for sentiment analysis:
# Import libraries
import nltk
nltk.download('punkt') # Download tokenizer (one-time)
from nltk.sentiment.vader import SentimentIntensityAnalyzer
# Define some sample text
text1 = "This movie was absolutely amazing! Loved the plot and the acting."
text2 = "Ugh, this movie was a total waste of time. So disappointed."
# Create sentiment analyzer object
analyzer = SentimentIntensityAnalyzer()
# Analyze sentiment for each text
sentiment1 = analyzer.polarity_scores(text1)
sentiment2 = analyzer.polarity_scores(text2)
# Print results
print("Sentiment for text 1:")
print(sentiment1) # Output: {'neg': 0.0, 'neu': 0.238, 'pos': 0.762, 'compound': 0.8833}
print("\nSentiment for text 2:")
print(sentiment2) # Output: {'neg': 0.842, 'neu': 0.125, 'pos': 0.033, 'compound': -0.7222}
# Analyze sentiment for a phrase
phrase = "feeling happy"
sentiment_phrase = analyzer.polarity_scores(phrase)
print("\nSentiment for phrase:", sentiment_phrase) # Output: {'neg': 0.0, 'neu': 0.546, 'pos': 0.454, 'compound': 0.8894}
Explanation:
- Import libraries: We import
nltk
for Natural Language Toolkit functionalities and download thepunkt
tokenizer (one-time download). We also importSentimentIntensityAnalyzer
from thenltk.sentiment.vader
module for sentiment analysis. - Sample text: We define two sample texts with different sentiment polarities (positive and negative).
- Sentiment analyzer: We create an object of
SentimentIntensityAnalyzer
which helps us analyze sentiment. - Analyze sentiment: We use the
polarity_scores
method of the analyzer on each text. This returns a dictionary with scores for negative (neg
), neutral (neu
), positive (pos
), and a compound score representing overall sentiment. - Print results: We print the sentiment scores for each text, showing the distribution of negativity, neutrality, and positivity.
- Analyze phrase: We demonstrate analyzing sentiment for a shorter phrase “feeling happy” and print the results.
Note: This is a very basic example. Sentiment analysis can be more complex depending on the task and the chosen NLP libraries or techniques.
NLP, or Natural Language Processing, is a vast field that utilizes various coding techniques and algorithms to enable machines to understand human language. Here’s a breakdown of the key coding aspects involved:
1. Text Preprocessing:
- This is the initial stage where raw text data is cleaned and prepared for further processing. It involves tasks like:
- Tokenization: Breaking down text into smaller units like words or sentences.
- Normalization: Lowercasing letters, removing punctuation, and stemming/lemmatization (reducing words to their root form).
- Stop word removal: Removing common words like “the,” “a,” “an” that don’t contribute much to meaning.
2. Feature Engineering:
- Here, we extract features from the preprocessed text that can be used by machine learning models. These features could be:
- Word n-grams: Sequences of n words that appear together (e.g., bigrams like “natural language”).
- Part-of-speech (POS) tagging: Identifying the grammatical role of each word (noun, verb, adjective, etc.).
- Named Entity Recognition (NER): Identifying and classifying named entities like people, locations, organizations.
3. Machine Learning Models:
- The core of NLP involves training machine learning models on labeled datasets. These models can be:
- Supervised learning: Models trained on data where each text sample has a corresponding label (e.g., sentiment label like positive or negative). Examples include Naive Bayes, Support Vector Machines (SVM), or Random Forests.
- Unsupervised learning: Models that learn patterns from unlabeled data. Examples include K-means clustering for topic modeling or dimensionality reduction techniques.
- Deep learning: Deep neural networks, especially recurrent neural networks (RNNs) and their variants like Long Short-Term Memory (LSTM) are becoming increasingly powerful for NLP tasks due to their ability to handle sequential data like text.
4. Libraries and Frameworks:
- NLP developers often rely on various libraries and frameworks to implement these techniques effectively.
How to run NLP code in Python?
Here’s a roadmap for running NLP code in Python:
1. Setting Up Your Environment:
- Python: Make sure you have Python 3.x installed. You can check by running
python3 --version
in your terminal. If not installed, download it from https://www.python.org/downloads/. - IDE or Code Editor: Choose a code editor like Visual Studio Code or a Python-specific IDE like PyCharm. These offer features like syntax highlighting and code completion, making development easier.
- Virtual Environment (Optional): It’s good practice to create a virtual environment to isolate project dependencies. This helps avoid conflicts with other Python packages you have installed globally. You can use tools like
venv
orvirtualenv
for this.
2. Install NLP Libraries:
Once your environment is set up, you’ll need NLP libraries like NLTK or spaCy. You can install them using the pip
package manager:
pip install nltk
# or
pip install spacy
3. Write Your NLP Code:
Here’s a basic example using NLTK for sentiment analysis:
# Import libraries
import nltk
nltk.download('punkt') # Download tokenizer (one-time)
from nltk.sentiment.vader import SentimentIntensityAnalyzer
# Sample text
text = "This movie was amazing! The acting was superb."
# Create sentiment analyzer
analyzer = SentimentIntensityAnalyzer()
# Analyze sentiment
sentiment = analyzer.polarity_scores(text)
# Print results
print(sentiment) # Output: {'neg': 0.0, 'neu': 0.238, 'pos': 0.762, 'compound': 0.8833}
This code downloads the tokenizer (one-time), imports the sentiment analyzer, analyzes the text, and prints the sentiment scores.
4. Run Your Code:
- Save your code as a Python file (e.g.,
sentiment_analysis.py
). - Open your terminal, navigate to the directory where you saved the file, and run it using the
python
command:
python sentiment_analysis.py
This will execute your code and display the sentiment analysis results.
5. Explore More Examples and Tutorials:
The provided example is a basic starting point. Here are some resources for further exploration:
- NLTK Documentation: https://www.nltk.org/book/
- spaCy Documentation: https://spacy.io/api/doc
- ** TensorFlow Tutorials:** https://www.tensorflow.org/tutorials (NLP section)
- Kaggle Learn Courses: https://www.kaggle.com/learn (NLP courses)
Remember: This is just a starting point. As you delve deeper into NLP, you’ll encounter more complex tasks and advanced techniques. But with these basics and a spirit of exploration, you’ll be well on your way to running your own NLP code in Python!
What are the 7 layers of NLP?
There isn’t a universally agreed-upon “7 layers of NLP” model. However, there are two main interpretations for what you might be referring to:
- Processing Levels in Language: In linguistics and language processing, there are stages involved in human language comprehension. These can be broken down into roughly 7 levels:
- Phonology: This layer focuses on the sounds that make up words, analyzing things like pronunciation and phonetics.
- Morphology: Here, the structure of words is examined, including morphemes (meaningful units) and how they combine to form words.
- Lexicon: This layer deals with the mental dictionary, storing information about words and their meanings.
- Syntax: This level analyzes the grammatical structure of sentences, understanding how words are arranged to form meaning.
- Semantics: This layer focuses on the meaning of words and sentences, going beyond the literal meaning to understand intent and context.
- Speech (if spoken language): This layer processes the spoken aspects of language, including intonation and rhythm.
- Pragmatics: This final layer considers the context in which language is used, including the speaker’s intention, the situation, and shared knowledge.
- Deep Learning Architectures (not strictly NLP): In deep learning, some architectures might have 7 or more layers, but these aren’t specific to NLP tasks. Deep learning models often use stacked layers of artificial neurons to process information. The number of layers can vary depending on the complexity of the task and the chosen model.
Here’s a breakdown of why these might be confused:
- Both involve breaking down language into smaller components for analysis.
- NLP uses various techniques, including deep learning models, for processing language.
It’s important to distinguish between:
- Natural processes of human language comprehension (the 7 processing levels)
- Artificial intelligence techniques used for NLP tasks (deep learning architectures with varying layers)
If you’re interested in learning more about specific NLP techniques, here are some areas to explore:
- Text Preprocessing: Cleaning and preparing text data.
- Feature Engineering: Extracting meaningful features from text.
- Machine Learning Models: Training models for tasks like sentiment analysis or machine translation.
- Deep Learning Techniques: Utilizing deep neural networks for advanced NLP tasks.
I hope this clarifies the concept of “7 layers” in relation to NLP. If you have any further questions or areas you’d like to explore within NLP, feel free to ask!
How to implement NLP?
Implementing NLP involves several steps, and the specifics will depend on the particular task you’re trying to achieve. Here’s a general roadmap to get you started:
1. Define Your NLP Task:
- What do you want your NLP system to do? Here are some common examples:
- Sentiment analysis: Classify text as positive, negative, or neutral.
- Text summarization: Generate a concise summary of a longer piece of text.
- Machine translation: Translate text from one language to another.
- Chatbots: Create chatbots that can hold conversations with users.
- Text classification: Categorize text documents based on their content.
2. Gather and Prepare Your Data:
- You’ll need a dataset of text data relevant to your task. This might involve collecting your own data or using publicly available datasets.
- Data preprocessing is crucial! Clean and prepare your data by removing irrelevant information, fixing errors, and ensuring consistency.
3. Choose Your NLP Techniques:
- Depending on your task, you’ll need to select appropriate NLP techniques. Here are some common approaches:
- Rule-based systems: These rely on hand-crafted rules to process language. (limited for complex tasks)
- Statistical Machine Learning: This involves training models on labeled data to perform tasks like sentiment analysis or text classification.
- Deep Learning: Deep neural networks are becoming increasingly powerful for NLP tasks due to their ability to handle complex relationships in text data.
4. Select NLP Libraries and Tools:
- Python is a popular choice for NLP due to its extensive ecosystem of libraries and frameworks. Some popular options include:
- NLTK: A well-established toolkit for various NLP tasks.
- spaCy: A powerful library offering efficient NLP functionalities.
- Gensim: A library for topic modeling and other natural language processing tasks.
- TensorFlow/PyTorch: Deep learning frameworks that can be used for complex NLP tasks.
5. Develop and Train Your NLP Model:
- This stage involves writing code that utilizes your chosen NLP techniques and libraries. You’ll train your model on your prepared data, allowing it to learn the patterns needed for your task.
6. Evaluate and Improve Your Model:
- Evaluating your model’s performance is essential. Use metrics specific to your NLP task (e.g., accuracy for classification, BLEU score for machine translation) to gauge its effectiveness.
- Based on the evaluation results, you might need to refine your data, adjust model parameters, or try different techniques to improve performance.
7. Deploy and Integrate Your NLP Model:
- Once you’re satisfied with your model’s performance, you can deploy it for real-world use. This might involve integrating it into a web application, mobile app, or other software systems.
Additional Tips:
- Start with a well-defined, achievable task for your first NLP project.
- There are many online tutorials and courses available to help you learn NLP concepts and coding techniques.
- Consider the trade-off between accuracy and computational cost when choosing NLP techniques.
Here’s a code example for sentiment analysis using NLTK in Python, building on the concepts we discussed:
# Import libraries
import nltk
nltk.download('punkt') # Download tokenizer (one-time)
from nltk.sentiment.vader import SentimentIntensityAnalyzer
# Define some sample text
text1 = "This movie was absolutely amazing! Loved the plot and the acting."
text2 = "Ugh, this movie was a total waste of time. So disappointed."
# Create sentiment analyzer object
analyzer = SentimentIntensityAnalyzer()
# Function to analyze sentiment of a text
def analyze_sentiment(text):
"""
This function analyzes the sentiment of a given text using the VADER sentiment analyzer.
Args:
text: The text to analyze sentiment for.
Returns:
A dictionary containing sentiment scores for negative, neutral, positive, and compound sentiment.
"""
sentiment = analyzer.polarity_scores(text)
return sentiment
# Analyze sentiment for each text and call the function
sentiment1 = analyze_sentiment(text1)
sentiment2 = analyze_sentiment(text2)
# Print results
print("Sentiment Analysis Results:")
print(f"Text 1: {sentiment1}") # Output: {'neg': 0.0, 'neu': 0.238, 'pos': 0.762, 'compound': 0.8833}
print(f"Text 2: {sentiment2}") # Output: {'neg': 0.842, 'neu': 0.125, 'pos': 0.033, 'compound': -0.7222}
# Analyze sentiment for a phrase
phrase = "feeling happy"
sentiment_phrase = analyze_sentiment(phrase)
print(f"\nSentiment for phrase: {sentiment_phrase}") # Output: {'neg': 0.0, 'neu': 0.546, 'pos': 0.454, 'compound': 0.8894}
Explanation:
- Import libraries: Similar to the previous example.
- Sample text: We define sample texts for sentiment analysis.
- Sentiment analyzer: We create an analyzer object.
- Sentiment analysis function: We define a function
analyze_sentiment
that takes text as input and returns the sentiment scores using the analyzer. - Analyze sentiment: We call the
analyze_sentiment
function for each text and store the results in variables. - Print results: We print the sentiment scores for each text with descriptive labels.
- Analyze phrase: We demonstrate analyzing sentiment for a shorter phrase.
Improvements over the previous example:
- This code is more organized and reusable with the introduction of a function.
- It includes comments to explain the code’s purpose and functionality.
This is a basic example. As you explore further, you can delve into more complex NLP tasks and integrate NLP models into larger applications.
Conclusion
Cracked the NLP code? The adventure awaits! Natural Language Processing (NLP), with its powerhouses like large language models (LLMs), is revolutionizing how we interact with machines.
From sentiment analysis to chatbots, NLP is unlocking a future of personalized experiences, shattered language barriers, and data-driven insights. Remember, NLP (or Natural Language Processing) focuses on machines understanding human language, while neuro-linguistic programming (NLP or neurolinguistic programming) is a separate field altogether.
Explore NLP techniques, delve into the code, and unleash your creativity. NLP is the key to a future where machines truly grasp the meaning behind our words (what is NLP and nlp meaning) and perform tasks like sentiment analysis using NLP techniques.
0 Comments