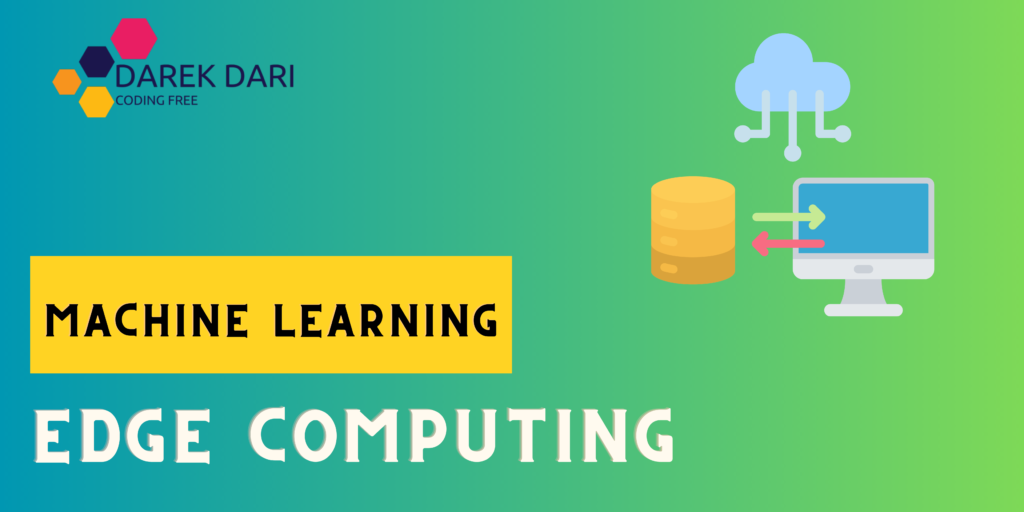
Table of Contents
Introduction
In today’s fast-paced technological world, two groundbreaking concepts, Edge Computing and Machine Learning, are coming together to transform the way we process information. Let’s explore the exciting synergy between these advancements and how they are revolutionizing our digital infrastructure.
Definition of Edge Computing
Edge Computing involves processing data closer to its source, reducing delays and improving response times. This shift from traditional cloud computing brings computing power directly to the “edge” of the network.
Overview of Machine Learning
Machine Learning, a subset of Artificial Intelligence, enables systems to learn from data and make decisions without explicit programming. Its adaptive nature makes it an ideal companion for emerging technologies.
Intersection of Machine Learning and Edge Computing
The convergence of Machine Learning and Edge Computing opens up exciting new possibilities for real-time decision-making, efficient data processing, and enhanced user experiences.
The Significance of Machine Learning in Edge Computing
Enhanced Decision-Making
By deploying Machine Learning algorithms at the edge, devices are empowered to make intelligent decisions independently. This leads to faster responses and improved efficiency across various applications.
Real-time Data Processing
Edge Computing, in conjunction with Machine Learning, facilitates the real-time processing of data. This is crucial for applications where immediate actions based on data insights are of utmost importance.
Improved Resource Allocation
Efficient resource allocation is vital for the success of any computing system. Machine Learning algorithms assist in dynamically allocating resources based on changing demands, optimizing overall performance.
Applications of Machine Learning in Edge Computing
Smart Cities
Machine Learning in Edge Computing is revolutionizing cities, transforming them into smart entities. Real-time analytics enable seamless operations in areas such as traffic management and waste disposal.
Healthcare
The combination of Machine Learning and Edge Computing is revolutionizing patient care in the healthcare sector. Wearable devices can monitor health parameters in real-time, allowing for timely interventions.
Autonomous Vehicles
The automotive industry is utilizing Machine Learning at the edge to enhance the capabilities of self-driving cars. This ensures quick decision-making on the road, ultimately improving safety.
Challenges and Solutions
Security Concerns
Securing data at the edge presents challenges. However, by implementing encryption, authentication, and robust security protocols, these concerns can be mitigated.
Resource Constraints
Edge devices often have limited resources. By optimizing machine learning models and utilizing edge-friendly algorithms, resource constraints can be effectively addressed.
Integration Issues
Integrating Machine Learning with existing systems can be complex. Clear integration strategies and compatibility checks are essential for a smooth implementation process.
Mitigation Strategies
Implementing layered security, continuous monitoring, and regular updates are key mitigation strategies for addressing challenges in Machine Learning at the edge.
Future Prospects
Advancements in Edge Machine Learning
As technology continues to evolve, Edge Machine Learning will witness advancements, leading to more sophisticated algorithms and improved efficiency.
Industry Adoption Trends
Industries are increasingly embracing Machine Learning at the edge. Understanding adoption trends is crucial for staying at the forefront of technological innovation.
Impact on Technological Landscape
The combined impact of Machine Learning and Edge Computing will reshape the technological landscape, influencing how businesses operate and deliver services.
Case Studies
Successful Implementations
Examining real-life examples of successful implementations provides valuable insights into the transformative power of Machine Learning at the edge across various industries.
Key Takeaways
By analyzing the lessons learned from past implementations, we can avoid common pitfalls and optimize future deployments of Machine Learning in edge computing.
Tips for Implementing Machine Learning in Edge Computing
Collecting Reliable Data
The success of Machine Learning heavily relies on the quality of data. Implementing robust data collection processes is crucial to ensure accurate and meaningful insights.
Fine-tuning Models
Optimizing machine learning models for edge devices is of utmost importance. Striking the right balance between accuracy and resource efficiency is key.
Collaboration between Edge and Cloud
Seamless collaboration between edge and cloud systems enhances the overall performance and scalability of Machine Learning applications.
Learn more
The Human Element
The Role of Human Input in Machine Learning
While machines learn from data, human input remains indispensable. Human expertise guides the development of algorithms and ensures ethical considerations are given priority.
Addressing Ethical Concerns
As Machine Learning becomes more integrated into our daily lives, it is crucial to address ethical concerns such as bias and privacy. Human oversight ensures responsible and ethical use of this technology.
Evolving Technologies
Edge Machine Learning Hardware
The progress of Edge Machine Learning heavily relies on the advancements in hardware technologies. Faster processors and specialized hardware contribute to better performance.
Software Innovations
Innovations in software, including algorithmic improvements and new programming paradigms, further enhance the capabilities of Machine Learning at the edge.
Collaborative Technologies
The collaboration between different technologies, such as Internet of Things (IoT) and Machine Learning, creates synergies that amplify the benefits of each.
Future Challenges and Opportunities
Anticipated Obstacles
Predicting and addressing future challenges, such as increased complexity and security threats, is vital for the continuous growth of Machine Learning in Edge Computing.
Emerging Possibilities
The ever-changing landscape brings forth new opportunities. Exploring emerging possibilities ensures staying ahead in the dynamic field of technology.
Conclusion
To sum up, the incorporation of Machine Learning into Edge Computing represents a major advancement in the capabilities of digital systems. The combination of these technologies not only improves efficiency but also paves the way for groundbreaking applications in different industries.
Is Machine Learning only applicable to large-scale industries?
Machine Learning has applications across industries of all sizes. Its versatility allows for implementation in various sectors.
How can businesses overcome security concerns when adopting Edge Machine Learning?
Businesses can address security concerns through encryption, authentication, and regular security audits.
What role does human input play in the development of Machine Learning algorithms?
Human input is essential for guiding the development of algorithms, ensuring ethical considerations, and addressing biases.
Are there any ethical considerations associated with Machine Learning in Edge Computing?
Yes, ethical considerations include issues of bias, privacy, and responsible use of technology.
What are the key factors for successful implementation of Machine Learning at the edge?
Robust data collection, model optimization, and collaboration between edge and cloud systems are key factors for successful implementation.
What do you mean by edge computing?
Edge computing involves processing data near the source of generation, reducing latency and improving response times.
What is edge computing vs cloud computing?
Cloud computing processes data in centralized servers, while edge computing processes data closer to its source, reducing latency.
What is edge in 5G?
In 5G, edge computing brings processing closer to the network edge, enhancing speed and efficiency for applications like IoT and augmented reality.
Is Edge Computing a Server?
Edge computing isn’t a single server but a decentralized network of servers or computing devices at the edge of a network.
What is edge computing vs cloud computing?
Edge computing processes data closer to its source, reducing latency, while cloud computing centralizes data processing in remote servers.
Is edge computing the future?
Many believe so! Edge computing’s efficiency and speed make it a promising technology for the future, especially with the increasing demand for real-time processing.
How does edge computing work?
Edge computing works by processing data near its origin, reducing the need for centralized servers. Devices at the “edge” of the network handle data processing, improving response times and overall efficiency.
0 Comments