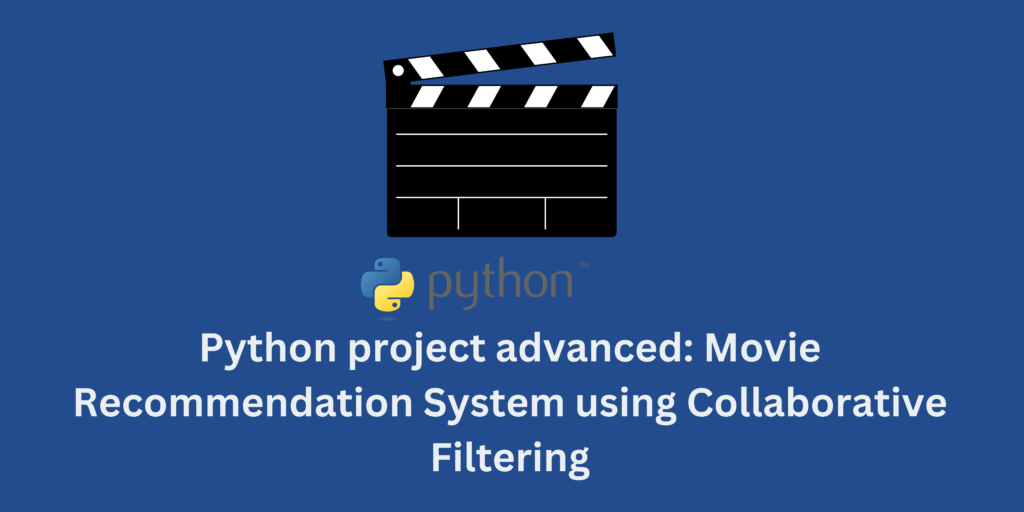
Python project advanced: Movie Recommendation System using Collaborative Filtering: here’s an advanced Python project with code. This project involves building a recommendation system using collaborative filtering.
Objective of Python project advanced
To build a movie recommendation system that suggests movies to users based on their previous ratings and the ratings of similar users.
Tools and Libraries Used:
- Python 3.6+
- Pandas
- Scikit-learn
Steps of the Python project advanced
- Load the data into a Pandas dataframe:
import pandas as pd
ratings_data = pd.read_csv('ratings.csv')
movies_data = pd.read_csv('movies.csv')
- Merge the two dataframes:
movie_ratings = pd.merge(ratings_data, movies_data, on='movieId')
- Group the movie ratings by user ID:
user_ratings = movie_ratings.groupby(['userId', 'title'])['rating'].max().unstack()
- Fill in missing ratings with 0:
user_ratings = user_ratings.fillna(0)
- Calculate the user similarity matrix using cosine similarity:
from sklearn.metrics.pairwise import cosine_similarity
user_similarity = cosine_similarity(user_ratings)
- Define a function to get similar users:
def get_similar_users(user_id, user_similarity_matrix, num_users=5):
user_similarity = user_similarity_matrix[user_id]
similar_users = user_similarity.argsort()[:-num_users-1:-1]
return similar_users
- Define a function to recommend movies:
def recommend_movies(user_id, user_similarity_matrix, user_ratings, num_recommendations=5):
similar_users = get_similar_users(user_id, user_similarity_matrix)
recommendations = []
for user in similar_users:
movies = user_ratings.iloc[user]
movies_not_watched = movies[movies == 0].index
for movie in movies_not_watched:
if movie in recommendations:
continue
rating = user_ratings.iloc[user][movie]
if rating > 3:
recommendations.append(movie)
if len(recommendations) == num_recommendations:
break
if len(recommendations) == num_recommendations:
break
return recommendations
- Test the recommendation system:
user_id = 1
recommendations = recommend_movies(user_id, user_similarity, user_ratings)
print(recommendations)
9. Deploy the recommendation system:
A Python web framework like Flask or Django can be used to deploy the recommendation system as a web application.
10. Improve the recommendation system:
- Using matrix factorization algorithms like Singular Value Decomposition (SVD) or Alternating Least Squares (ALS).
- Incorporating contextual information like the time of day or the user’s location.
- Using deep learning models like neural networks to learn non-linear relationships between users and movies.
3 Comments
Binance账户 · March 19, 2024 at 12:32 pm
Your point of view caught my eye and was very interesting. Thanks. I have a question for you.
Учетная запись в binance · April 28, 2024 at 3:40 am
Thanks for sharing. I read many of your blog posts, cool, your blog is very good.
binance Registrierung · July 5, 2024 at 8:00 am
Your article helped me a lot, is there any more related content? Thanks!