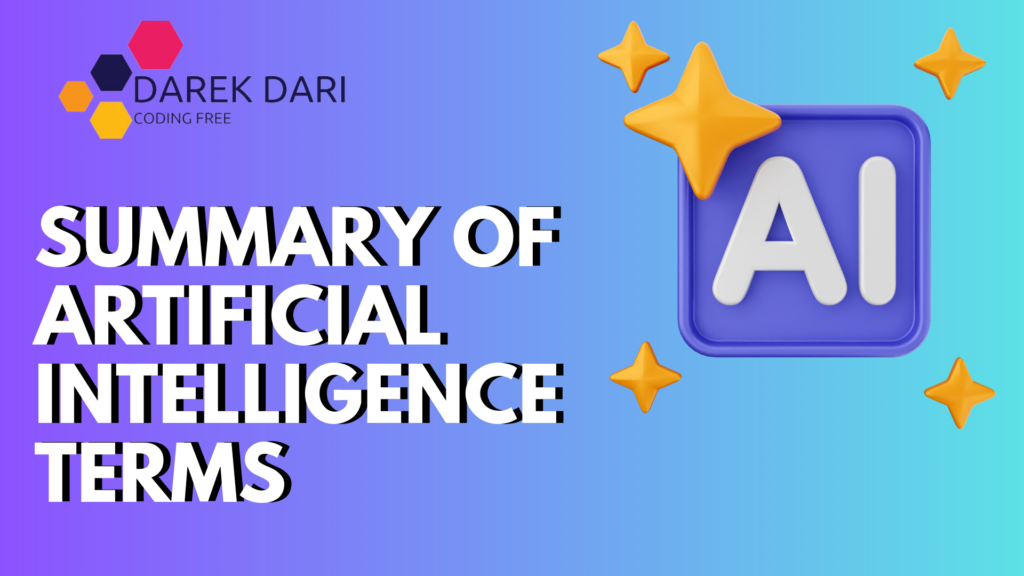
Table of Contents
Hey there, tech enthusiasts! 🌟 Welcome to our latest dive into the fascinating world of Artificial Intelligence (AI)! As we gear up for 2024, understanding the key terms in AI has never been more exciting and crucial.
Whether you’re just getting started or looking to brush up on the latest trends, buckle up as we unpack the essential AI terms that are shaping the future.
From machine learning and neural networks to cutting-edge innovations like GANs and transfer learning, let’s demystify these concepts and discover how they’re revolutionizing everything from healthcare to gaming. Ready to embark on this AI adventure? Let’s dive in!
What is Machine Learning?
Machine learning is like teaching computers to learn from experience. Instead of giving them step-by-step instructions, we feed them lots of data, and they figure out patterns and make predictions all on their own. It’s how your favorite apps get smarter and more helpful over time!
What is Deep Learning?
Deep learning is a subset of machine learning that focuses on using neural networks with many layers (hence “deep”) to analyze and learn from large amounts of data.
It’s inspired by the way the human brain works, allowing computers to recognize patterns, make decisions, and even understand complex concepts like images, speech, and language. Think of it as a more advanced and powerful version of machine learning!
What is Artificial Intelligence?
AI, or artificial intelligence, refers to the simulation of human intelligence in computers and other machines.
This means creating systems that can perform tasks typically requiring human intelligence, such as understanding language, recognizing patterns, solving problems, and making decisions.
AI encompasses a variety of techniques, including machine learning and deep learning, to enable machines to learn from experience, adapt to new inputs, and perform human-like tasks.
What is Neural Networks?
Neural networks are a fundamental component of artificial intelligence modeled after the human brain’s structure and function. They consist of interconnected nodes, or neurons, organized in layers. Information flows through these layers, where each neuron processes data and passes it on to the next layer.
Neural networks can learn patterns and relationships within data, enabling tasks like image recognition, language translation, and more complex decision-making processes in AI systems.
What is Natural Language Processing (NLP)?
Natural Language Processing (NLP) is a field within artificial intelligence that aims to help computers comprehend, interpret, and produce human language in a meaningful and practical manner. It involves the communication between computers and humans using natural language.
NLP covers various tasks like text categorization, sentiment analysis, language translation, named entity recognition, and speech recognition. Its uses include chatbots, language translation tools, text summarization, and sentiment analysis for social media monitoring, among other things.
What is Computer Vision?
Computer vision is a branch of artificial intelligence that empowers computers to analyze and comprehend the visual realm through digital images or videos. This field focuses on creating algorithms and methods that enable machines to derive insights from visual data, like images or videos.
Tasks in computer vision encompass object detection, image categorization, facial identification, motion tracking, and scene comprehension. The uses of computer vision span from self-driving cars and medical image interpretation to augmented reality and quality assurance in production.
What is Reinforcement Learning?
In reinforcement learning, an agent learns how to make decisions by engaging with its surroundings. The goal is to increase rewards over time by choosing actions that result in positive results.
Unlike supervised learning, which uses labeled data, or unsupervised learning, which identifies patterns in unlabeled data, reinforcement learning relies on trial and error.
Through feedback in the form of rewards or punishments, the agent can develop effective strategies for tasks like gaming, robotics, and resource allocation.
What is Supervised Learning?
Supervised learning is a type of machine learning where the model is trained on a labeled dataset. Labeled data means that each input example is paired with the correct output or target. The goal of supervised learning is for the model to learn the mapping between input features and the corresponding output labels.
During training, the model adjusts its parameters to minimize the difference between its predictions and the actual labels in the training data. This process allows the model to generalize to new, unseen data and make accurate predictions or classifications.
ai artificial intelligence cast
ai film cast
ai movie cast
ai artificial intelligence actors
ai the movie cast
artificial intelligence in education
artificial intelligence and education
artificial intelligence for education
artificial intelligence on education
Supervised learning is widely used in various applications, such as image classification, spam detection, sentiment analysis, and medical diagnosis, where labeled data is available for training and evaluating the model’s performance.
What is Unsupervised Learning?
Unsupervised learning is a type of machine learning where the model is trained on data that lacks explicit labels or predefined outcomes. Instead of learning from labeled examples, unsupervised learning algorithms explore the structure and patterns within the data to extract meaningful insights or representations.
ai for education
ai in education
education and artificial intelligence
education artificial intelligence
The primary goal of unsupervised learning is to uncover hidden patterns or structures within the data without prior knowledge of what the output should look like. Common tasks in unsupervised learning include clustering, where similar data points are grouped together, and dimensionality reduction, which simplifies the data while preserving its key features.
Unsupervised learning is used in applications such as customer segmentation, anomaly detection, and recommendation systems, where the data is unstructured or lacks labeled examples for training a supervised model.
top ai stocks
top artificial intelligence stocks
best ai apps
etf artificial intelligence
artificial intelligence movie cast
artificial intelligence cast
a i movie cast
cast of ai artificial intelligence
a i artificial intelligence cast
What is Artificial Neural Networks (ANN)?
Artificial Neural Networks (ANN) are computer systems that mimic the neural structure of the human brain. They are made up of interconnected nodes (neurons) that analyze data and acquire patterns through training algorithms.
ANNs play a crucial role in machine learning, as they can identify patterns, make predictions, and tackle intricate issues in fields such as image recognition and natural language processing.
ai and healthcare
ai for healthcare
ai in healthcare
artificial general intelligence in healthcare
artificial intelligence in healthcare:
artificial intelligence for healthcare
artificial intelligence definition
What is Convolutional Neural Networks (CNN)?
CNNs, or Convolutional Neural Networks, are a type of artificial neural network specifically designed to process grid-like data, such as images or audio.
Inspired by the visual cortex of animals, CNNs use convolutional layers to extract features from inputs, making them ideal for tasks like image recognition, object detection, and image classification.
Through convolutional operations, pooling layers, and fully connected layers, CNNs can automatically learn hierarchical representations of data and effectively capture spatial hierarchies and patterns, making them popular in computer vision applications.
artificial intelligence companies
ai companies
artificial-intelligence companies
artificial general intelligence jobs
ai jobs
artificial intelligence job
artificial intelligence in healthcare
What is Recurrent Neural Networks (RNN)?
Recurrent Neural Networks (RNNs) are a type of artificial neural network designed to handle sequential data, where the order of inputs matters. Unlike feedforward neural networks, RNNs have connections that form a directed cycle, allowing them to exhibit dynamic temporal behavior.
RNNs are structured to process sequences of inputs one element at a time, maintaining an internal state that captures information about what has been processed so far. This capability makes them well-suited for tasks such as speech recognition, natural language processing, and time series prediction.
However, traditional RNNs can struggle with capturing long-term dependencies due to vanishing or exploding gradient problems. As a result, more advanced variants like Long Short-Term Memory (LSTM) networks and Gated Recurrent Units (GRUs) have been developed to address these issues and improve the learning and retention of long-term dependencies in sequential data.
ai etf
ai stocks to buy
a i meaning
artificial intelligence movie
artificial intelligence steven spielberg
ai: artificial intelligence
artificial intelligence.movie
inteligencia artificial movie
What is Generative Adversarial Networks (GAN)?
Generative Adversarial Networks (GANs) are a type of machine learning framework introduced by Ian Goodfellow and his colleagues in 2014. GANs consist of two neural networks: the generator and the discriminator, which are trained simultaneously in a competitive manner.
- Generator: This network generates new data instances, such as images, based on random input (latent) vectors.
- Discriminator: This network evaluates the generated images, determining whether they are real (from the training data) or fake (generated by the generator).
The key idea behind GANs is that the generator improves over time to produce increasingly realistic data by trying to fool the discriminator, while the discriminator gets better at distinguishing real data from generated data. This adversarial training process leads to the generator producing high-quality outputs that are indistinguishable from real data.
GANs have revolutionized areas such as image generation, image editing, and data synthesis, and they continue to be a vibrant area of research in machine learning and artificial intelligence.
what is artificial intelligence
what is the ai
artificial intelligence app
ai app
ai apps
app artificial intelligence
artificial intelligence apps
artificial intelligence etf
What is Transfer Learning?
Transfer learning is the process of using a pre-trained model that has learned from a large dataset and adjusting it to a new task with a smaller dataset.
By utilizing the knowledge stored in the pre-trained model’s weights, transfer learning enables quicker training and frequently leads to improved performance for the new task, even when data is scarce.
This method is particularly beneficial in fields such as computer vision and natural language processing, where pre-trained models can accelerate the learning process and enhance accuracy.
artificial intelligence newspaper
ai movie
artificial intelligence 2001 movie
ai artificial intelligence the movie
artificial intelligence what is
what is an ai
What is Robotics?
Robotics is a field that covers a wide range of applications, from industrial robots used in manufacturing and assembly lines to autonomous robots in agriculture, healthcare, space exploration, and even domestic settings.
It combines elements of mechanical engineering, electrical engineering, computer science, and artificial intelligence to create systems capable of sensing their environment, making decisions, and executing actions to accomplish specific tasks efficiently and safely.
artificial-intelligence
what is ai
what is a ai
artificial intelligence news
ai news
news about artificial intelligence
What is Expert Systems?
Expert Systems are software programs that imitate the decision-making skills of human specialists in specific areas. They utilize information stored in databases or rule-based systems to examine data, address issues, or offer guidance.
These systems are applied in various sectors like healthcare, finance, and engineering to simplify decision-making procedures and guarantee reliable, precise results rooted in expert knowledge and regulations.
Conclusion
As we conclude our journey exploring the latest AI terms of 2024, it’s evident that this field is progressing rapidly.
From the fundamental concepts like machine learning and neural networks to the advanced applications such as GANs and transfer learning, AI is constantly pushing the boundaries of what is achievable. Looking forward, one thing is certain: keeping up-to-date with these essential terms will be crucial in navigating the exciting advancements that lie ahead in AI.
artificial intelligence
ai
artificial intelligence stocks
ai stocks
artificial general intelligence stocks
artificial intelligence stock
artificial intelligence:
Whether you are an experienced professional or a curious novice, continue to delve deeper, keep expanding your knowledge, and embrace the transformative impact of Artificial Intelligence in shaping our future. Here’s to a future where AI continues to motivate and innovate!
0 Comments